“Generative” music is a term that has become ubiquitous in the modular synthesizer community. You might have seen long “generative ambient” videos that feature moody lighting and decorative succulents or “generative techno” tracks with LEDs pulsing in a darkened room. But what exactly is “generative” about this music, and how can we get started making our own generative systems?
A Brief Introduction to Generative Processes in Art
The term “Generative Music” was apparently coined by Brian Eno in the mid 1990s, but the ideas that gave rise to generative music have their roots in a constellation of ideas that have been increasingly explored by artists and musicians throughout the 20th and 21st century. Although Eno's definition of “Generative Music” covers just a narrow range of generative methods, numerous techniques exist within this realm. These are frequently referred to by a variety of related (but not necessarily synonymous) terms such as “algorithmic music,” “aleatoric music,” “stochastic music,” and “process art.”
Since industrialization, and particularly in the computer age, the automation of common activities by opaque systems has become common in our lives. For some artists the definition of a creative activity has similarly expanded to include creative tasks that involve organizing systems which themselves create work of art. Recent breakthroughs in deep learning and large language models have paved the way for artificial intelligence to create convincing simulations or imitations of creativity, a concept which is now a familiar trope.
But the idea that the job of the artist could be to create a process that creates the art, rather than creating the art directly, has deep roots, especially in visual and sculptural media. Sol LeWitt's Wall Drawings notably foreshadow many themes prevalent in modern generative art. Beginning in 1968, the American artist Sol LeWitt created over 1,300 Wall Drawings. Each drawing consists of an instruction rather than a specific visual work. The instructions provide a specific set of guidelines for crafting a mural-sized artwork. Intriguingly, the execution of these drawings doesn't necessitate the artist's hand; others can bring the instructions to life. This flexibility means that numerous Sol LeWitt creations have emerged even posthumously, after his passing in 2007. True to their concept, the directives for these drawings are typically succinct, leaning heavily on geometric procedures. For instance, Wall Drawing #33 prescribes a one-inch grid of black squares, within which any amalgamation of vertical black lines, horizontal yellow lines, right-diagonal red lines, or left-diagonal blue lines can be drawn with colored pencil. The stark juxtaposition between the concise nature of these instructions and the resulting expansive and visually complex murals is likely one element in their enduring influence and appeal. [Ed: see the YouTube video above, which documents an installation of Wall Drawing #1136 at the Scottish National Gallery of Modern Art.]
In music, we can trace experiments with generative techniques even earlier. Greek composer and early electronic music pioneer Iannis Xenakis experienced the cacophony of gunfire while fighting with the Greek resistance during and after World War II. In listening to this sound, Xenakis realized that with a sufficiently high density of sound events, a new sound emerged—no longer individual gunshots, but rather a density of noise in which the timing of individual sounds was unimportant. This revelation steered Xenakis towards a novel approach in music composition, utilizing math and in particular probability theory, to create dense and otherworldly textures, blending both noise and tone. His experiments with generative algorithms explored a remarkable diversity of generative techniques including game theory, Markov chains, random walks, Brownian motion, and statistical models for natural phenomena, such as the dispersion of gaseous molecules.
During the 20th century, algorithmic composition primarily focused on using generative techniques to create fixed, notated musical scores intended for performers. In these instances, the term "generative" refers to the algorithmic procedure employed to formulate the score. However, some composers explored techniques that yield a unique composition with each performance.
As early as the 1950s composers such as Karlheinz Stockhausen, Earle Brown, and Morton Feldman were exploring musical scores with modular sections that could be re-rearranged, ensuring that each performance would be slightly different. This approach to composition—where certain elements of the music were determined according to chance operation—became known as aleatoric music, although the term is used broadly for several different approaches. This includes music which is composed through random processes, music which is strictly notated but in which certain elements are left open to the discretion of the performer, and music in which certain aspects of the notation are indeterminate or require the performer to perform chance procedures as part of the performance.
Although better known for his “silent” work 4’33”, in which the performer sits in silence for the entirety of the work, American composer John Cage was incredibly influential on the development of aleatoric approaches to music. Many of his works include some random or generative process used either in the creation of the score or the actual performance of the work. These techniques include works generated from consulting the I Ching (an ancient Chinese text and method of divination), works generated from imperfections in the sheets of paper, works generated from the positions of astronomical bodies in star charts, and works derived procedurally from other pre-existing books and musical works.
While Sol LeWitt’s Wall Drawings and Xenakis's stochastic composition techniques exemplify aleatoric works, their generative nature is distinct. In these instances, the indeterminacy arises from the score or the performer's interpretation of it. This contrasts with the contemporary definition of generative music, which is largely perceived as music originating from a live process. Whether this process is stochastic or deterministic, it progresses with limited human intervention.
Complexity from Simple Origins
Algorithmic and probabilistic processes have been a staple of contemporary music for decades, but the 1990s marked a transformative era for technologically-inclined artists. The advent of the personal computer, along with the rise of the internet, provided unprecedented tools and platforms for creation and dissemination. This period saw a surge in artists leveraging technology not only as an instrument but also as a partner in the creative process. The newfound accessibility to powerful computing allowed more artists to explore computational processes at home rather than in rarified research institutions, while the internet fostered a global community where artists could share, collaborate, and push the boundaries of what was previously thought possible in the realm of music.
For the new avant garde there were new influences: simple computer games, screensavers, and of course new approaches to digital sound synthesis. An art school graduate with an awareness of experimental music and art rock, Brian Eno was perfectly positioned to capture and express this zeitgeist.
In a 1996 lecture at the Imagination Conference in San Francisco, Brian Eno reflected on generative music as music which derives complex patterns from simple rules. Interestingly, Eno uses a simple graphic illustration as a metaphor for generative music, evoking patterns reminiscent of minimalist visual arts and even Sol LeWitt.
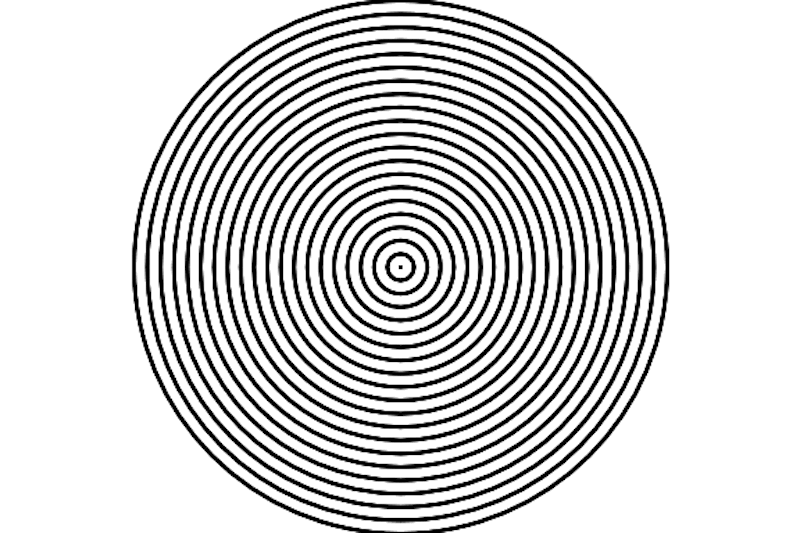
[Above: an example of a simple moiré pattern.]
A moiré pattern is an optical illusion resulting from interference patterns formed when two patterns of lines are overlapped and moved across each other, similar to the patterns that occur when two sets of ripples meet on the surface of a smooth pond. In a moiré pattern, the pattern of lines is simple and the movement of lines relative to each other is predictable. But the human eye sees a complex and evolving mosaic as a result of the intersection of these movements.
For Eno, these patterns represent a visual corollary to generative music. They are a visual proof of concept. Interesting complexity can arise from the intersection of simple processes.
Auditory moiré pattern existed prior to Brian Eno’s usage of the term. In fact in the same lecture Eno also references It’s Gonna Rain, a tape-loop composition by Steve Reich in which a short recording of a phrase spoken by a Pentecostal preacher is looped at two slightly different speeds simultaneously. The overlapping loops and slight displacement of timing creates an unbelievably rich and evolving acoustic tapestry as words and background noises echo and blur.
[Above: Eno's seminal Discreet Music, as remastered in 2004.]
Eno’s early music leaned heavily on these types of asynchronous tape loops. As early as (No Pussyfooting) in 1973 and Discreet Music in 1975, Eno was experimenting with recording into two tape machines, each of which was also recording into each other. In this way sound was re-recorded in a feedback loop between the machines, layering sounds into expansive soundscapes and presaging numerous contemporary ambient tracks that use similar feedback and re-recording techniques.
Eno's seminal album, Ambient 1: Music for Airports (1978), notably incorporates tracks centered on tape loops with a more deterministic approach. Within each track, discrete notes or brief musical segments are overlaid with unhurried loops that seldom align perfectly. Take the third track, "1/2", as an example: it meshes at least eight succinct piano segments, each composed of one to four notes, looped at intervals ranging from seventeen to thirty-eight seconds. Due to their asynchronous nature, the loops ensure that, across numerous iterations, the audience won't encounter a configuration identical to the outset. It's a system devoid of randomness, being wholly deterministic: one can precisely predict its evolution from the initial state and loop durations. Yet, the ensuing "moiré pattern" captures the serendipity and weight of unforeseen coincidence.
While both Brian Eno and early minimalist composers were interested in the contrast between the simplicity of a process and the complexity of the musical result, in the mid '90s, Brian Eno’s interest seems to have moved towards algorithms and the way algorithmic music continuously changes and evolves over time without the need for direct human intervention. This fascination with the interplay between defined rules and emergent complexity led Eno to explore simple computer algorithms and even generative screensavers, which were highly optimized to minimize their computational requirements on early desktop computers.
One such algorithm that Eno spent a great deal of time observing was Conway's Game of Life, a “zero-player game” invented by British mathematician John Horton Conway in 1970. A form of cellular automata, Game of Life models living cells as squares on a grid. In each round of the game, squares either live, die, or multiply based on the number of other cells in neighboring squares. The rules are minimal (e.g. “A live cell with fewer than two neighbors dies”), but the game famously produces vivid and spontaneous patterns that are deterministically related to the starting state of the grid. Populations bloom and suddenly collapse. Small groups of cells get stuck in never-ending loops. Periodic eddies appear that move around the board in a seemingly directed way.
What is fascinating about an algorithm like Life is that such simple rules lead to a system that really feels like an organic process. After thousands of generations of activity, Life still feels like a living, changing system. This is also a feature of generative music. Instead of following a predetermined, linear composition, generative music embraces the beauty of spontaneous patterns that emerge from simple rules and the ever-changing interplay between them. This allows for continuous evolution and adaptation, mirroring the dynamic nature of the natural world.
Eno explicitly contrasts this conception of “generative” music with earlier styles of “static” art:
“Classical music, like classical architecture, like many other classical forms, specifies an entity in advance and then builds it. Generative music doesn't do that, it specifies a set of rules and then lets them make the thing.”
In Eno’s later work the explicitly algorithmic aspects of this idea have come to the fore. This has included, to date, six generative music applications released in the Apple App Store in collaboration with Peter Chilvers, including Air (2009), which is a live generative reworking of Music for Airports in the form of an app.
Early Synths and Generative Music
Generative music for synthesizers got a somewhat slow start. Following consumer demand, American synth designer Robert Moog had added a keyboard to synthesizer prototypes as early as 1964. While this made the instrument more accessible for musicians who were reassured by a familiar control interface, many early musical usage of the synthesizers tended to treat the instrument as a kind of novel electric organ, like the Mellotron, exploiting its possibilities more for its unique timbral potential rather than as a transformative tool for musical exploration and innovation. The Moog synthesizer can be heard in this capacity on several albums from the '60s such as The Beatles’ Abbey Road, where it can be heard primarily as a sound effect or adding a bit of color doubling keyboards and guitars.
Contrary to the Moog philosophy, Moog-competitor Donald Buchla favored unfamiliar control interfaces. A California-born engineer with a degree in physics, Buchla strove to avoid reference to familiar music instruments. Instead of keyboards, Buchla’s 100 Series modular synthesizer, produced beginning around 1965 through the early 1970s, featured keyboard-like arrays of capacitive touch plates which were less likely to suggest a familiar usage pattern and encouraged experimentation.
[Above: detail of an early Buchla 112 Touch Controlled Voltage Source—part of the Series 100 prototype housed at Mills College. Image via Sarah Belle Reid.]
For early modular artists who fell into Don Buchla’s orbit, the lack of a traditional keyboard became almost a credo. It was a symbol of this new instrument’s utopian potential and hallmark of a new kind of virtuosity. For artists like Suzanne Ciani, who became acolytes of the Buchla 200 system in the 1970s, the extensive implementation of voltage control offered a completely new way of thinking about sound:
“It was about the sound moving in space and the sound moving with a lot of controls, in other words, I didn't have a base sound that I loved because the sound would go from base to crystal! In a second, less than a second. We were dealing more with the movement of the sound from low to high range, moving around the room, the timbre moving, everything was moving. Because [Buchla] had control voltage inputs for everything!” – Suzanne Ciani interview with Inertia Movement Cloudcast (Berlin, 2016)
While many modular artists may have received their generative inspiration from Don Buchla, it was the Serge Synthesizer that made it accessible to weekend warriors and independent artists.
The creation of Serge Tcherepnin, a colleague of Morton Subotnick’s at CalArts, the Serge system is today known for its banana cable patch cords, its iconic designs, such as the Dual Universal Slope Generator (of which Make Noise Maths is a distant cousin), and for its famously arcane and rarified generative patching techniques.
While studying Don Buchla’s designs for the 100 Series synthesizer in the late '60s, Tcherepnin realized that each Buchla module was essentially a collection of smaller circuits, combined, abstracted, and presented under the pretense of a single function. From the beginning, Tcherepnin was determined to modularize each of these subsystems, while still following Buchla’s preference for patchability and avoiding keyboards and other familiar controllers. The result was a highly customizable set of circuits. Instead of a VCO that contained within it an oscillator, a wave shaper, a rectifier, and several comparators, Tcherepnin’s designs break these subsystems out as separate components, each with their own numerous patch points and control voltages.
[Above: detail of a late (c. 1977/78) "paperface" Serge modular system at CalArts. Image via Sarah Belle Reid.]
This shift marked a particularly modern interpretation of patch programmability, removing rigid, preconceived musical functions traditionally associated with individual modules and letting users build these functions from scratch. Instead of being restricted to the functions of an envelope generator or an oscillator, there is a "universal slope" generator. New names for modules mark this shift: the Triple Bi-Direction Router, the Peak and Trough, Triple Comparator, the Analog Shift Register, the Smooth and Stepped Generator. These functions all do something to voltage. But what that voltage does artistically is completely up to the user.
Serge brought generative synthesis to a much wider audience. Serge systems were cheaper than alternatives from Buchla, Moog, ARP, and others—being offered as early as 1973 as DIY kits for just $600. Commonly referred to as “the people’s synthesizer,” early Serge systems quickly became a popular choice for younger artists and hobbyists and were acquired by several research institutions.
The Serge design philosophy had an immediate impact on music too. Early Serge albums from the 1970s break new ground with generativity. A landmark album from 1978, David Chesworth's album The Unattended Serge, is a collection of excerpts from a single 24-hour recording of the Serge system at La Trobe University in Melbourne, Australia, which was left to run without human intervention. While Buchla artists like Morton Subotnick and Suzanne Ciani had previously ventured into the realm of generative music, The Unattended Serge represents a distinct evolution of the genre. The composition is the patch. The work’s structure is simply the outcome of the fluctuation of voltage in the system.
Synthesizers as Information Systems
From the earliest synthesizers to the present day, parallel approaches to synthesis persist. Synthesizers don’t have to be used in a generative way. They can be treated like an acoustic instrument, or they can be used like a digital audio workstation (DAW) to create musique concrète, with tightly controlled grids of sound events precisely positioned in space and time. But synthesizers are particularly well suited to the creation of generative systems because, like simple computer programs, they have the ability to maintain and update an internal state.
Although we’re familiar with the idea that computers, including digital synthesizers, store information, the idea that analog synthesizers also store and manipulate information might be puzzling. Information in the digital world is represented as a sequence of discrete values. Binary is one example. Binary is a two-level logic—ones and zeros. While certain functions in a synthesizer can be represented as finite-state machines, synthesizers represent state (control voltage) as both quantized and unquantized voltage. This voltage is used both as audio and information, allowing the user to set the level on a VCA or trigger an envelope, for example. That information, the state of voltage at a particular time, can be saved, evaluated, updated, randomized, and even fed back on itself to modulate its own state.
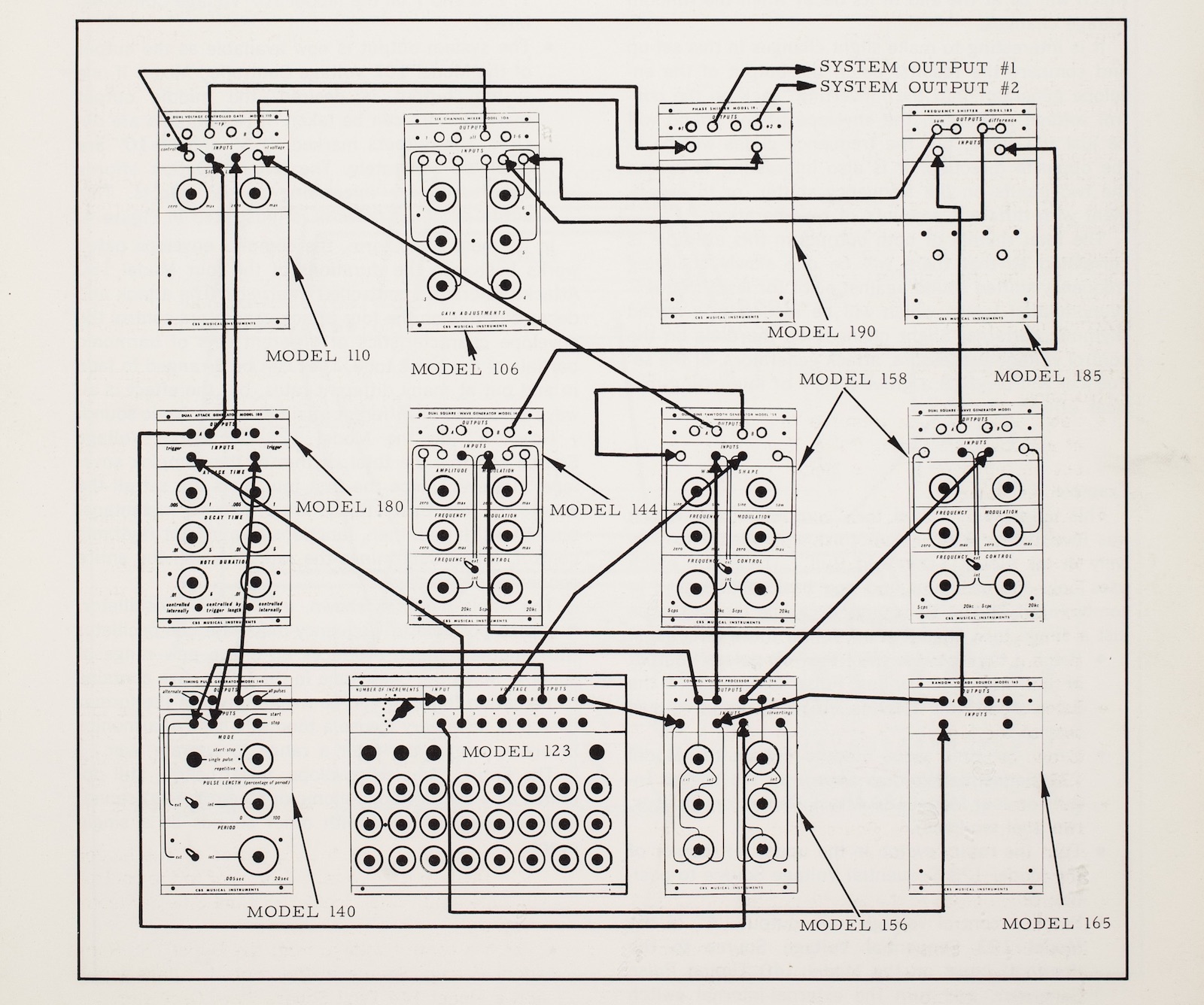
[Above: detail of a complex patch from Hubert Howe's user manual for the Buchla 100 system, prepared for CBS in the late 1960s/early 1970s.]
What makes hardware synthesizers, and in particular modular synthesizers, so uniquely well suited to generativity is the ease by which state can be modulated and self modulated. The structure of a patchable synthesizer does not suggest any single evaluation orders. Audio and information can move laterally or feed back on itself.
Common generative patches take full advantage of this “flat” routing scheme by moving voltage and control information out of simple linear channels to derive unique side effects from unrelated sources. They often employ multiple asynchronous processes that intertwine and modulate one another. Commonly, these patches feature loops or shifts in levels where the voltage from either an initial or advanced stage in the signal chain is repurposed. This might involve modulating an earlier stage (control voltage feedback), or influencing a later stage. For instance, a randomly-sampled voltage might be used both to control a sequence length and redirected to control a late-stage VCA or filter. The correlation of these unrelated features leads to unexpected musical structures, and these interconnections and self-modulating mechanisms give generative patches their dynamic and evolving intricacy.
Krell et al.
Since the 1960s, a number of generative patches have been documented and even become well known (Allen Strange's “Dream Machine,” Douglas Leedy's “Entropical Paradise,” etc.). These patches serve as inspiration and as a useful exercise in learning to patch more generativity, as well as being artistic works in their own right. A useful exercise is to replicate well-documented patches (or some part of these patches) and to examine what aspects of the patch are generative and how the system generates variation and evaluation over time.
Probably the most famous generative patch, the Krell Muzak patch, was first created by Todd Barton in 2012. Although the original patch was demonstrated with a Buchla 200e series modular synthesizer, many (if not most) imitators have sought to replicate the patch on Eurorack systems. This has led to some confusion and several documented versions of the patch are claimed to be the definitive diagram of the Krell patch. Regardless of these differences however, the core patch contains a few main elements that serve as a useful introduction to some of the most common generative techniques.
The heart of the Krell patch is a looping attack-decay envelope whose attack and decay times are randomized. This is the element of the patch that gives it a Krell patch its distinctive expressive quality.
[Above: Todd Barton introducing a variation on the original Krell patch concept, focusing on Tiptop Audio's Buchla 200-inspired Eurorack modules—and centered specifically around the 266t Source of Uncertainty.]
The attack and decay portions of the envelope may be modulated with any voltage source, such as random voltage source, LFO, etc. But, as in Barton's original patch, they are most commonly modulated by two separate and unsynchronized looping attack-decay envelopes. The attack-decay times on these modulating envelopes may themselves also be modulated. This setup, with an envelope modulated by two other unsynchronized envelopes, creates a very complex, although deterministic, pattern where the listener is unlikely to hear the same envelope twice in any reasonable span of time.
The end of cycle (EOC) triggers from the main envelope are used to control at least two pitch (V/8) control voltage generators which may be quantized or unquantized. One control voltage is used to set the pitch of a main oscillator, and the other voltage is used to set the pitch of a modulation oscillator which modules the main oscillator, usually via frequency modulation. Although the patch can be built without oscillator modulation, the addition of this feature functions like a random timbre generator, making the patch much more musical.
Finally, the main oscillator passes through a VCA which is also opened and closed by the main envelope. This creates the distinct randomized bell-like tones.
In Barton's original patch, there are also several filters after the VCA stage, which may also be randomized in some way or can be modulated by either the main envelope or by either of the modulation envelopes, leading to a more dynamic patch where the filter moves independent of oscillator pitch.
Although the Krell patch is highly dependent on randomized parameters, notice that almost everything in the patch is related in some way to the main envelope. So while the core generative strategy is structured around cycling asynchronous envelopes or random voltage, moments of change or evolution are always correlated in interesting ways, which leads to a very spontaneous and almost choral effect.
Next we’ll look at another powerful generative technique which depends less on coincidence while still generating surprising change over time.
Information Feedback
Let’s examine a specific technique which is at the core of a whole class of generative patches. I like to refer to this as the “information feedback” technique. We’ll look at a simple patch to illustrate the point, but the idea can be applied to many different types of patches. It’s not limited to hardware synthesizers either. We could build this type of generative patch in a digital music programming environment. As a concept, it's an exciting starting point for creativity, immediately situating the instrument in a generative space where the outcome of a patch is often surprising, even when the patch is fully deterministic.
While many musicians are familiar with the idea that audio feedback can be used creatively, the idea that the information in a synthesizer—represented by control voltage—can be fed back in a loop and used to control itself, might be a riveting, if not slightly dizzying, revelation.
In order for information feedback to be effective, we need a circuit that can evaluate the state of voltage and respond conditionally to that information, either comparing it to other voltage or comparing it to an internal criteria. There are several types of circuits that can be used to evaluate the state of control voltage. For example, analog logic modules can be used to compare two voltages and output a third voltage based on the state of the input voltages. Analog-to-digital converters, such as Xaoc Devices Drezno do something similar. But probably the most useful type of circuit for patching “if this, then that”-type logic would have to be the comparator circuit.
Patching Conditional Statements With Comparators
A simple comparator is a circuit that “goes high” (outputs a gate) based on whether an input voltage is over or under an arbitrary level. If our comparator is set to listen for 1 volt, the output of the comparator would be low (off) when the input signal was 0.2 volts and high (on) when the input voltage was 1.5 volts, for example. One way to think of a comparator is as a way to convert an analog signal to a digital signal. You can also think of a comparator as a way to create conditional logic with voltage. If a certain voltage condition exists, some other event is triggered. What exactly you do with that information is up to you.
A slight variation on the simple comparator, the window comparator, is a circuit that outputs a gate when the input voltage is within a voltage range. For example a window comparator could be set to go high when input voltage is between 1.1 volts (lower bound) and 1.2 volts (upper bound), and would be low at all other times. Of course in most comparators the range or boundaries can themselves also be modulated with control voltage.
Comparators and window comparators have a myriad of uses in generative music. Having a synthesizer control itself to produce surprising states that evolve over time is much easier when we have the ability to create conditional logic with voltage. A particularly interesting use case for a window comparator is found when we combine the comparator with quantized pitch control voltage.
The Self-Modulated Sequencer Patch
A simple example of an information feedback patch involves controlling a sequencer with a circuit that evaluates the output of that sequencer. (Or in simple terms, patching a sequencer to control itself.)
It’s hard to pin down a specific origin for this patch. Versions of it have no doubt been explored for years, especially in Serge systems. A similar patch is explained in a video by Michael Manning in 2021 (embedded above), although in Manning’s video, the comparator is used to derive generative beats which are linked to specific pitch voltages rather than to control the sequencer itself. Control voltage feedback has been discussed on the ModWiggler modular synthesizer forum since at least 2012. Regardless of its origins, the core of this patch consists of a sequencer or other voltage “memory” which is controlled in part through a comparator or window comparator which is itself evaluating the output of that sequencer.
Note that this is a feedback patch but not of a typical kind. There is voltage feedback, but not audio feedback. The pitch control voltage can be used for anything later in the chain. It can control an oscillator, filter cutoff, etc. The key generative technique here is the feedback of data, represented as voltage, to control the continued production of new structurally-complex voltage states.
In Michael Mannings patch, the Joranalogue Compare 2 window comparator is used to evaluate both of the two sequences coming out of the Intellijel Metropolix sequencer. Drums are triggered based on whether the pitch control voltage from the sequencer is within the comparator’s window. This leads to beats which are intricately linked to the pitch sequence. When the sequence changes, so do the beats. Since Metropolix is a two-channel sequencer, and Joranalogue Compare 2 is a two-channel window comparator with logic gates between the left and right sides, it’s possible to generate complex generative states from the coincidental intersection of two asynchronous sequences. When both sequences are simultaneously within their windows, both sides of the comparator go high, triggering a rare “AND” state, and so on.
Here unsynchronised sequences take the place of tape loops, but the principle is similar to Brian Eno’s early tape-loop compositions. The generative principle is simple and deterministic: sequences of unequal lengths and events which are triggered when voltage from both sequences falls within a range.
We can take this idea a step further however by controlling the sequencer itself with the window comparator. (A simple comparator is also possible, but a window comparator is particularly useful since it allows for specific pitch ranges, and even single quantized pitches, to be used as conditionals.) When a certain pitch or range of pitches triggers the window comparator, we use the gate to trigger some change to the sequence itself, which is itself analyzed by the comparator, and so on.
How we control the sequencer with the window comparator depends to some extent on the design of the sequencer. If the sequencer allows for it, we could use the window comparator to—for example—transpose, rotate, invert, or randomize the sequence. Some sequencers allow even more advanced options. The Frap Tools Usta allows for voltage-controlled pattern recall from the sequencer’s digital memory. The Orthogonal Devices ER-101/102 allows stochastic algorithms reminiscent of Xenakis, including randomization of pitches within a range and pitch jitter by specific intervals.
But if the sequencer/voltage memory can’t be modulated, there are other ways to still achieve generative data feedback. We could use the window comparator to control another module which is modifying the sequence in some way. For example the window comparator could control the offset or octave transposition of pitch CV. Or the window comparator could control a slew applied to the sequence.
Conclusion
The advent of AI-generated images and large language models has brought the artistic merit and ethical considerations of generative art to the forefront of contemporary discourse. But this is not a new debate. AI is simply the modern continuation of a long history of experimentation with generative art, a journey that stretches back over a century.
Generativity doesn't exist in absolutes. Rather, it is a gradient with varying degrees, spanning from rigid control to complete autonomy in the artistic process. The idea that generativity can coexist with control might diverge from the way Brian Eno originally conceptualized the term, but the 20th century was rife with artists and theorists who ventured into this domain. They explored generativity as it related not just to deliberate artistic creation, but also as it echoed the naturally occurring sounds and processes present in ambient environments. These experiments weren't just about creating art, but about recognizing and emphasizing the inherent generative qualities of the world around us.
Generative art, in all its forms, challenges our perceptions of authorship and originality. From “prepared” field recordings—which capture sounds artificially staged in pre-existing acoustic environments—to sophisticated AI algorithms, the exploration of generative art continues to manifest the dance between chaos and order, serendipity and design, which exists just beneath the surface of creativity. Synthesizers are just one, albeit deep and fascinating, manifestation of this artistic approach.